In a timely conversation on DEI data, Phil Shrader of One Model and Season Chapman and Yuli Lopez of Culture Curated shed light on the importance of diversity and inclusion data analytics.
While strides have been made in leveraging people analytics to propel the DEI movement forward, they reveal a stark reality: The journey towards achieving comprehensive diversity data standards is far from over.
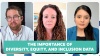
What’s Missing and What’s Present in Your DEI Data?
As we delve deeper into the complexities of gathering DEI data, it becomes evident that significant gaps in what is collected hinder progress toward truly inclusive environments. Critical areas needing attention and improvement include:
Performance reviews and gender bias
Season Chapman highlighted a concerning statistic: In a significant study, 66% of women received negative personality-related feedback in performance reviews, compared to less than 1% of men. (Source)
This discrepancy not only exposes a gender bias but underscores the need for a more nuanced approach to evaluating performance and collecting performance data. By systematically analyzing both the written and verbal components of reviews, organizations could begin to identify biases entrenched in their evaluation processes.
Ageism and Strength-Based Diversity
The often overlooked dimension of age bias, dubbed by the American Psychological Association as 'the last socially acceptable prejudice,’ highlights a gap in DEI initiatives’ predominant focus on racial and gender bias.
Season also highlighted the tendency to emphasize weaknesses rather than strengths in organizational cultures. Incorporating strength-based analytics into DEI strategies could revolutionize how talents are matched with roles, fostering a more inclusive and productive workplace environment.
Do you track and measure these 4 diversity metrics?
Awareness of DEI Data Bias Types
The above examples and many others highlight the significant potential for bias in data and data collection. Bias can exist within current data due to a variety of factors, including but not limited to:
- Historical bias can exist when past data, such as male-dominated hiring patterns, favors men over women for certain roles.
- Representation bias can occur when data used to train an algorithm may over- or underrepresent some groups. An example of this is found with facial recognition tools that produce higher error rates for certain groups.
- Measurement bias can happen when data that is collected disproportionately values behaviors or achievements that are more accessible to a particular group.
- Algorithmic bias can result when algorithms use their own predictions to make future decisions, which can replicate and even amplify existing biases in the dataset.
It’s important to note that there’s no such thing as completely bias-free data. (Source)
But we must seek to mitigate bias in our analytics by choosing effective technology, increasing our awareness of how it occurs, and applying safeguards.
3 Key Considerations in Advancing DEI Through Analytics
Exploring the landscape of diversity data reveals three pivotal areas essential for effective DEI strategies:
- Accurately interpreting and applying DEI data: To achieve this, organizations can use advanced analytics and visualization tools that enable stakeholders to see beyond the surface-level numbers. This enables them to identify underlying patterns and insights that drive targeted, effective DEI interventions.
- Ensuring data collection methods capture the full diversity of an organization: This involves developing and implementing data collection strategies that are inclusive of all identities and experiences, thus mitigating biases that could skew the understanding of the organization's diversity landscape.
- Addressing privacy, confidentiality, and bias in data and algorithms: Organizations should establish multidisciplinary ethics committees that regularly review data collection, analysis practices, and algorithmic decisions for biases. This oversight ensures continuous alignment with ethical standards and promotes fairness and equity in all AI-driven DEI decisions.
How One Model Supports DEI Initiatives
Modern enterprises must do more than just track hiring metrics; they need to deeply analyze diversity data to drive genuine improvements. Leveraging people analytics software like One Model enables organizations to reduce bias and harness insights for crafting policies that foster long-lasting diversity and inclusion.
Our clients use One Model's powerful analytics to visualize and monitor their DEI journey, establishing robust strategies that not only report but actively shape a more inclusive workplace.